Every day 1,300 scientists and 700 research institutes collect massive amounts of data. Their goal is track down cancer. Jan Komorowski, a bioinformatician involved in the project, in conversation with Monika Redzisz
Monika Redzisz: What is Pan-Cancer?
Jan Komorowski: It is a global project I and 1,300 other scientists and 700 research institutes from around the world are involved in. Pan-Cancer is primarily about sharing data and making all results available to other participants. There is a rule according to which creators of data are given priority to publish them, but other participants can use them earlier and publish the results after the embargo expires. It’s a very sound approach. It is of benefit to everyone.
Pan-Cancer is as important to oncology as the Large Hadron Collider is to physics – we generate huge amounts of data which are later used by many teams. There is only one major difference: in the case of the Collider data are generated in one location whereas in our case they are created in many places.
Hadn’t it been possible for scientists to share information or create a shared database before the project was launched?
It’s not so simple. In Pan-Cancer we are divided into several dozens of working groups but we are bound by the same procedure. We all do research in the same way, but even if we use the same sequencers, we have to correct data to eliminate the effects of local conditions affecting data values.
Until recently, studies on cancer were conducted in small teams using small data sets, but it turned out that in order to be able to conduct comprehensive research on diseases consisting in DNA mutations, you need an enormous amount of data which cannot be collected and processed by a single team. That is why many research centers joined their forces to launch cooperation projects. Statistics has its own demands.
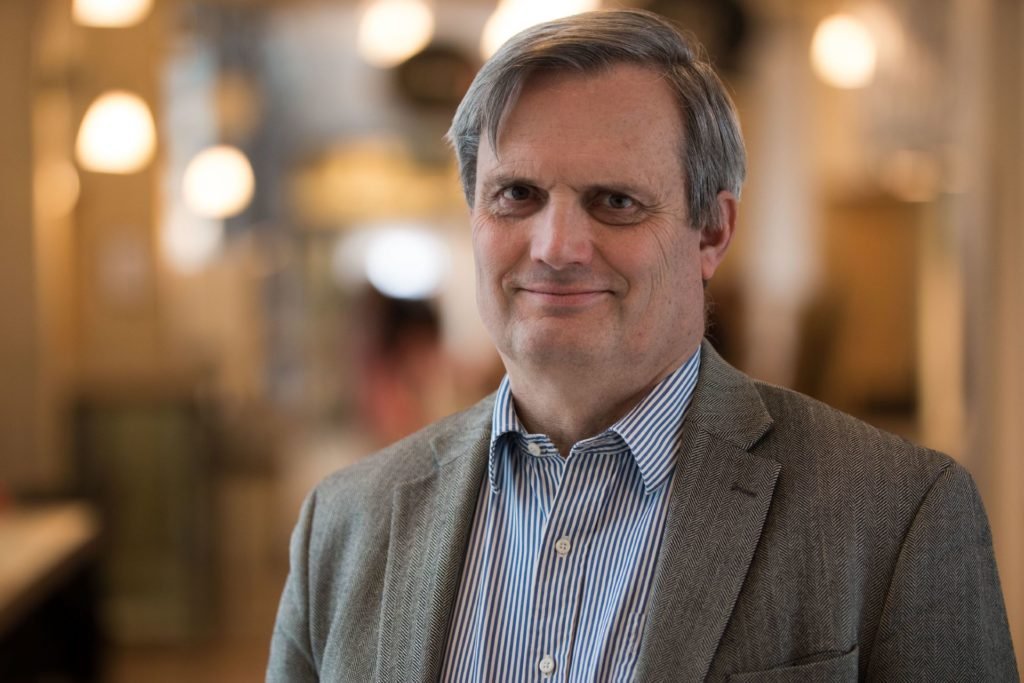
What have you managed to achieve?
We have analyzed complete genetic codes of over 2,600 tumors. The main conclusion drawn from the results we have obtained is that the cancer genome is finite, i.e. it undergoes repetitive mutations depending on the type of tumor.
We have discovered that many tumors are characterized by 4 or 5 main mutations which control their development. You can say that we have presented the most accurate image of tumors known to date.
Let me briefly explain how they are formed. A gene is a part of the DNA genome that consists of the starting sequence, also known as the promoter, and the coding sequence, or gene body. A tumor is a result of a major mutation in the genome, and not necessarily in a gene. Until recently, the focus was primarily on coding sequence mutations; however, now we know that carcinogenic mutations also occur outside the gene body in the so-called non-coding regions. One of Pan-Cancer’s goals was to analyze the non-coding regions found in gene regulatory elements. The most important result to date is confirmation that some tumors are caused by mutations in regulatory regions.
We have also discovered that, in general, there is no single mutation that would cause cancer. A number of mutations are needed for cancer to develop. And that’s the reason why testing them with statistical methods focused only on one variable does not bring good results.
What impact will it have on the oncological practice?
We will be able to develop new anti-cancer drugs. At present we have several medicines that can effectively counter certain mutations in the coding regions. Pan-Cancer results specify new places where drugs used to counter mutations in the regulatory region could be applied.
As a member of the bioinformatics community I can tell you that we are not picky – we are going to “eat” whatever we can digest. We care about signs and symptoms. Whether a patient shows signs of cancer or type 2 diabetes, we use similar methods to respond
Another extremely interesting result includes the fact that it is possible to determine the age of a given mutation. About 20 percent of the mutations are formed over many years or even decades before cancer can be diagnosed. We are talking here mainly about acquired and not hereditary mutations. Acquired as a consequence of smoking, alcohol, UV exposure, chemicals and so on. Put simply, we will be able to tell: this person has had mutation A for 10 years, mutation B was formed 3 years ago and if mutation C appears in the body, cancer will develop. We will be able to monitor the oncogenesis process. We will have tools to better understand the development of tumors, which will translate into more accurate diagnostics.
What does the division of labor between the teams consist in? Does your team deal with a specific type of tumor?
Liver cancer and leukemia are the diseases that have provided us with the largest amount of data, but as a member of the bioinformatics community I can tell you that we are not picky – we are going to “eat” whatever we can digest. We care about signs and symptoms. Whether a patient shows signs of cancer or type 2 diabetes, we use similar methods to respond. Our analyses are based on bioinformatic methods used to deal with big data sets and on machine learning methods. This is how we have discovered significant mutations in the genomic regulatory regions.
To oversimplify, until recently most research in biology or medicine based on the following pattern: a hypothesis that a certain gene gives rise to a given disease, an experiment consisting in modification of the gene, and observation of the results. Hypothesis, experiment, hypothesis verification.
What about today?
Today research can be conducted in a different manner. We don’t have to come up with a precise hypothesis. We can create data for several dozen or maybe hundreds of specific tumor samples and compare them with healthy tissue, called controls, of the same patients. We are looking for differences between samples and controls; we are discovering genes and, thanks to our methods, their combinations and values of the level of regulation (the level of regulation is a relative measurement of the degree of gene activation between the sample and the control), which we have not suspected of being involved in these processes.
We use supervised machine learning to discover not only genes, but also their regulation levels connected, in this case, with the oncogenesis characteristics. Traditional methods would focus on the genes giving a specific character to samples, but present in more significant or less significant groups; we provide a fuller description of gene sets. Obviously, the process also allows us to confirm previously specified genes.
The role of computer scientists in medicine is becoming a key to success.
Yes. Today, an IT expert participating in a biomedical project has to be a partner and not just a technical assistant. They can’t work with data after an experiment is complete; they have to take part in creating them from the very beginning. My laboratory consists mainly of PhD students specialized in computer science who perform very interesting analyses in cooperation with biomedical experts.
What do you use machine learning for in Pan-Cancer?
We have used it chiefly to generate data. We are now moving on to the next stage in which we will be able to use supervised learning to detect significant mutations in regulatory regions, for example. My research is usually supported by two pillars: we do research based on machine learning, but at the same time we use standard statistical methods. The first application of machine learning in this project consists in learning how to combine different types of data to effectively detect significant mutations in non-coding regions.
How long have you been dealing with artificial intelligence?
Since I was a student. We were the first year to study computer science in then newly established Institute of Informatics at the University of Warsaw. Back then I was dealing with natural language processing. I got involved in machine learning many years later. I was fascinated by the elegance of the rough set theory of professor Zdzisław Pawlak, which is not only based on strong mathematical foundations, but also allows to create classifiers whose operation can be explained.
How was artificial intelligence in Poland perceived at that time? How many people were interested in it?
There were probably only a handful of persons interested in AI in our narrow circle of IT specialists. The field was certainly popularized by professor Stanisław Waligórski, a scientist recognized by American researchers and an expert in a programming language called LISP. But the most prominent mathematicians dealing with functional analysis or topology looked at artificial intelligence pityingly. It wasn’t mocked at but no one would go into ruptures over it either.
She said: “Incredible. This man knows more about that gene than my postdocs”. Obviously, that wasn’t me. It was my program which gleaned the information from 10 million articles
After I defended my thesis in 1976 I was admitted to PhD studies and in 1977 I was awarded a Fulbright scholarship, which made it possible for me to go to the Massachusetts Institute of Technology. Unfortunately, the university rector told me that I could go only if I joined the communist party. I remember exactly what I replied to him: “Sooner or later I will go there anyway!”
And I did. Five years later I was at Harvard University and MIT, but I wasn’t a doctoral student. I was an Assistant Professor. However, before that, I had gone to Sweden, where I had defended my doctoral thesis under the supervision of professor Erik Sandewall, who was one of the world’s leading scientists in artificial intelligence. My doctoral thesis introduced the concept of partial evaluation in logic programming and one of the languages of artificial intelligence, i.e. Prolog. Partial evaluation, later referred to as partial deduction, is a simplification of programs due to partially known input data. It turned out that the principle of partial evaluation in logic programming applies not only to compilation, but also to optimization of queries in databases and constitutes a form of machine learning. The results of my doctoral thesis were presented at one of the most prestigious conferences on programming languages and my dissertation has been cited ever since. Soon after, I received a number of job offers at American universities, e.g. Harvard University, where I spent almost seven years, including the time at the Artificial Intelligence Laboratory at MIT and Harvard Medical School.
Why did you decide to dedicate yourself to bioinformatics?
I worked at Harvard Medical School for two years. Back then I collaborated with biomedical specialists and I had the opportunity to closely analyze the applications of computer science in medicine. After returning to Europe, in Trondheim, Norway, I took up interest in the rough set theory of professor Zdzisław Pawlak, which is a Polish approach to machine learning. Together with professor Andrzej Skowron from the University of Warsaw we created a system called ROSETTA, which implemented rough set algorithms. At some point, we were the fourth machine learning system in the world.
It was about that time when the technological revolution in molecular biology and medicine started. We saw the development of first mass sequencing methods with the use of DNA microarrays. A DNA microarray is a microscopic chip with DNA fragments called probes which correspond to mRNA sequences produced from genes we wish to analyze. A DNA microarray can be compared to a gene database. A sample with genetic material is a query for the database: which of the genes in my sample, and, to be more precise, which mRNA of these genes have a relatively higher or lower expression (Editor’s note: the expression of genes is a process allowing to decode information contained in a gene and transcribe it to gene products, e.g. RNA or protein). Answers to these questions are possible thanks to hybridization, i.e. combining the sequences from the probe with complementary fragments of the sample. The hybridization signal is detected with a fluorescent dye and marked in red for increased gene expression and in green for reduced gene expression. By comparing tumor DNA and healthy tissue DNA, we are able to identify what differences in gene expression are characteristic for the oncogenesis process.
We were one of the first teams in the world to apply supervised learning in experimental data obtained with the use of cDNA. We published our papers in renowned magazines such as “Genome Research”, “Nature Genetics” and “Bioinformatics” and managed to obtain significant funds for bioinformatics research, although at that time we called it computational biology.
Was that the turning point?
Yes. That was the moment when I decided I should retrain. I learned one very important thing: if you want to work on interdisciplinary projects, you must have exceptional scientific achievements in at least one of the disciplines. I took on bioinformatics as a renowned computer scientist.
In 2002 Upssala University offered me a position of Head of Bioinformatics Department. That was the first professorship in bioinformatics in Sweden. I also became the Director of the Linnaeus Center for Bioinformatics.
You were one of the persons who created that field of study.
To a certain extent. I’ve been dealing with the genomic data analysis since early 2000s. We have managed to comprehensively analyze the areas where transcription factors are bound to DNA, to regulatory regions. We searched for information based on keywords in the titles or abstracts. Our rate of correct answers was 60 percent on average, which was enough to quickly get the information we needed. Our paper on that subject was published in “Nature Genetics”. I remember someone asking me during a lecture to show them how it works. I typed the name of a certain central gene in angiogenesis taking place during the oncogenesis process (Editor’s note: carcinogenic angiogenesis is a process of creating new blood vessels occurring in the case of many malignant tumors) and after a short while the program returned a network of 20 genes. The professor who specialized in angiogenesis said: “Incredible. This man knows more about that gene than my postdocs”. Obviously, that wasn’t me. It was my program which gleaned the information from 10 million articles that could be found in the PubMed database.
Computers will replace humans in all sorts of tasks and they will perform them better than we ever would. But even though we have cars and planes, we have never quit cycling or running
Soon after we were invited to participate in prestigious projects. One of them was ENCODE – Encyclopedia of DNA Elements. The results of our studies were published in “Nature”, which translated into several thousand citations. Getting involved in the Pan-Cancer project was a natural course of things. We were invited to take part in it and we accepted the offer.
The project continues. It shows how important collaboration is.
Yes. I would encourage my Polish colleagues specializing in bioinformatics and biomedicine to participate in international projects as much as possible. In Poland we have a lot of great bioinformatics and biomedicine specialists, which is an excellent opportunity for our country to step up its presence in the international scientific community. With the appropriate funding and support for young people, Poland might become a country with huge potential in this field. Maybe the National Science Center should encourage the researchers to take part in programs similar to Pan-Cancer?
Unfortunately, today young researchers prefer to work abroad.
That’s true but Sweden has the same problem: the most talented Swedish researchers are moving to the USA or Great Britain, because that’s where the best centers are located. Unfortunately, in Poland we still haven’t broken with the hierarchical style of work: young PhD scientists publish their papers in collaboration with their supervisors. You won’t see that in Sweden. If a young person is awarded a grant, then he or she is the main author of what they do. In Poland young researchers are not allowed to be fully independent. That’s why they get discouraged.
When is Pan-Cancer going to end? Do you think it will evolve in any way?
Nothing has been decided yet. It’s a stepping stone for other projects. Other groups will probably make use of our data to further develop their ideas. I think that Pan-Cancer will become a set of data available to all researchers. There will be a huge open database that might be connected to other local bases. For example, in the UK, we have the project of 100,000 genomes supplemented with information from the medical case history. Connecting all those databases to Pan-Cancer would result in making the diagnoses much faster and more accurate. For example, we will be able to analyze in detail the so-called rare diseases that affect 6,000 to 8,000 people a year in Great Britain, if I remember correctly. At present, such diseases are extremely difficult to categorize.
Artificial intelligence is playing an increasingly important role in diagnostics. Do you think it might compete with doctors and scientists?
Nowadays artificial intelligence is often equated with machine learning. But they are not the same thing. Yann LeCun, a co-recipient of the Turing Award, which is considered an unofficial Nobel Prize in computer science, said that machine learning might be called “intelligent” only if it was able to infer. In fact, artificial intelligence is only our goal. We can see it on the horizon and we are going in that direction.
Some time ago Patrick Winston, director of the MIT Artificial Intelligence Laboratory, was asked if he was afraid of computers and robots becoming more intelligent than humans, to which he replied: “No, I’m not afraid. I am intelligent enough.” There is no doubt that computers will replace humans in all sorts of tasks and they will perform them better than we ever would. But even though we have cars and planes, we have never quit cycling or running.
We will be automating various aspects of human cognition and reasoning, but a human being can very easily adapt to different situations and has incredible self-improvement skills. I think it’s going to be healthy competition.
*Jan Komorowski, PhD – professor in bioinformatics at Uppsala University and a visiting professor in the Institute of Computer Science, Polish Academy of Sciences. He worked at Harvard University and in the Artificial Intelligence Laboratory at MIT. In 2002 he became the head of the Bioinformatics Department and was appointed the director of the Linnaeus Center for Bioinformatics. In 2019 he chaired “Artificial Intelligence for Life Sciences” conference in Sweden. He has published his papers and articles in “Nature Genetics”, “Genome Research”, “Nature”, “Nucleid Acid Research”, „Nature Communications”, “Bioinformatics” and “Scientific Reports”. He has been cited 15,345 times.